Data loss refers to an event in which important data is lost to the enterprise, such as in a ransomware attack. Data loss prevention (DLP) focuses on preventing illicit transfer of data outside organizational boundaries.
Data loss prevention is a set of tools and processes used to ensure that sensitive data is not lost, misused, or accessed by unauthorized users. DLP enforces remediation with alerts, encryption, and other protective actions to prevent end users from accidentally or maliciously sharing data that could put the organization at risk.
DLP is also used to describe software products that help a network administrator control what data end users can transfer. So, DLP products use business rules to classify and protect confidential and critical information so that unauthorized users cannot accidentally or maliciously share data, which would put the organization at risk. Also, some DLP tools can also be used to filter data streams on the corporate network and protect data in motion.
DLP technologies are broadly divided into two categories:
- Enterprise DLP: These solutions are comprehensive and packaged in agent software for desktops and servers, physical and virtual appliances for monitoring networks and email traffic, or soft appliances for data discovery.
- Integrated DLP: This solution is limited to secure web gateways (SWGs), secure email gateways (SEGs), email encryption products, enterprise content management (ECM) platforms, data classification tools, data discovery tools and cloud access security brokers (CASBs).
Source: skyhighnetworks.com
DLP products may also be referred to as data leak prevention, information loss prevention or extrusion prevention products.
Source: whatis.techtarget.com
What goals do organizations use DLP to achieve?
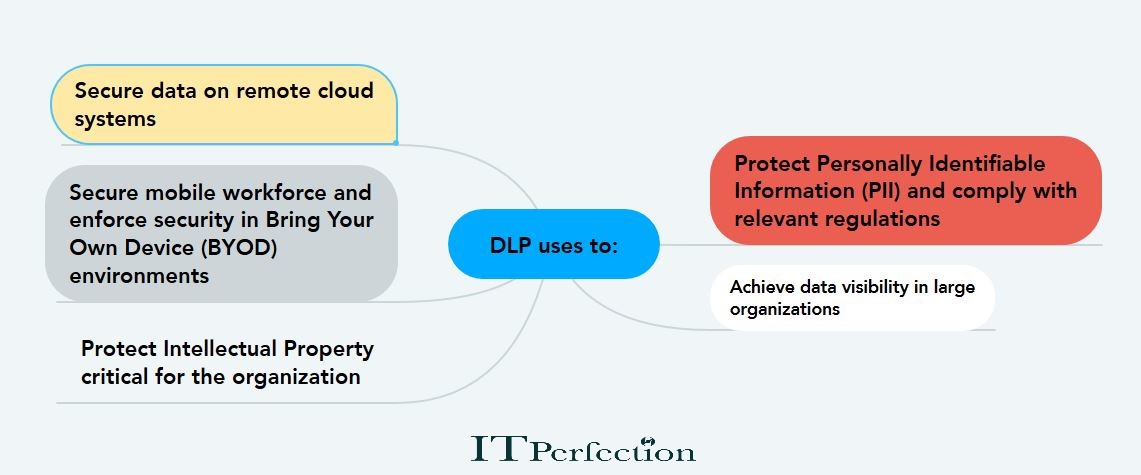
Source: imperva.com
Data Loss Prevention Best Practices
- Prioritize Data: You must to decide which data would cause the biggest problem if it were stolen. DLP should start with the most valuable or sensitive data that is likely to be targeted by attackers.
- Identifying roles and responsibilities: Clearly define the roles and responsibilities of the individuals involved in your organization’s DLP program.
- Classify the data: A simple, scalable approach is to classify data by context. Applying persistent classification tags to the data allows organizations to track their use. Content inspection is also useful. It examines data to identify regular expressions, such as keywords. Content inspection often comes with pre-configured rules for PCI, PII, and other standards.
- Working together with business unit heads to define the DLP policies that will govern organization’s data: This will help ensure that the different business units are aware of the policies in place and how they might be impacted.
- Understanding when data is at risk: There are different risks associated with data distributed to user devices or shared with partners, customers and the supply chain. A robust DLP program must account for the mobility of data and when data is at risk.
- Defining success metrics: You must define success metrics and share reporting with business leaders. Determine the key performance indicators (KPIs) you should measure and monitor them closely to determine the success of your DLP program and areas of improvement.
- Monitor data in motion: Organizations need to monitor data in motion to gain visibility into what’s happening to their sensitive data and to determine the scope of the issues that their DLP strategy should address.
- Communicate and develop controls: Controls can target common behaviors that most line managers would agree are risky. As the DLP program matures, organizations can develop more granular, fine-tuned controls to reduce specific risks.
- Train employees: Employees often don’t recognize that their actions can result in data loss and will do better when educated. Advanced DLP solutions offer user prompting to inform employees of data use that may violate company policy or increase risk.
- Rollout: Some organizations will repeat these steps with an expanded data set or extend data identification and classification to enable fine-tuned data controls. By initially focusing on securing a subset of the most critical data, DLP is simpler to implement and manage.
Source: proofpoint.com and digitalguardian.com
How Does DLP Work?
Understanding the differences between content awareness and contextual analysis is essential to comprehend any DLP solution in its entirety.
- Content awareness: It involves capturing the envelope and peering inside it to analyze the content
- Context: It includes external factors such as header, size, format, etc., anything that doesn’t include the content of the letter.
Anyway there are multiple content analysis techniques which can be used to trigger policy violations, including:
- Rule-Based/Regular Expressions: this is the most common analysis technique used in DLP. This technique involves an engines analyzing content for specific rules such as 16-digit credit card numbers, 9-digit US social security numbers, etc. This technique is an excellent first-pass filter.
- Database Fingerprinting (Exact Data Matching): This mechanism looks at exact matches from a database dump or live database.
- Exact File Matching: In this technique, file contents are not analyzed; however, the hashes of files are matches against exact fingerprints. This approach does not work for files with multiple similar but not identical versions.
- Partial Document Matching: Looks for complete or partial match on specific files such as multiple versions of a form that have been filled out by different users.
- Conceptual/Lexicon: Using a combination of dictionaries, rules, etc., these policies can alert on completely unstructured ideas that defy simple categorization.
- Statistical Analysis: This technique uses machine learning or other statistical methods such as Bayesian analysis to trigger policy violations in secure content. Requires a large volume of data to scan from, the bigger the better, and else prone to false positives and negatives.
- Pre-built categories: Pre-built categories with rules and dictionaries for common types of sensitive data, such as credit card numbers/PCI-DSS protection, HIPAA, etc.
Today when evaluating DLP solutions, pay close attention to the types of patterns detected by each solution against a real corpus of sensitive data to confirm the accuracy of its content engine.
Source: skyhighnetworks.com
Types of DLP Technologies
- DLP for data in use: These DLP technologies defined as data that is being actively processed by an application or an endpoint.
- DLP for data in motion: Encryption plays a large role in this step. Email security is also critical since so much business communication goes through this channel.
- DLP for data at rest: DLP technologies protect data residing in a variety of storage mediums, including the cloud. DLP can place controls to make sure that only authorized users are accessing the data and to track their access in case it is leaked or stolen.
This section is abbreviated from cisco.com
What’s Data Leak?
Data leakage is the unauthorized transmission of data from within an organization to an external destination or recipient. Data leakage threats usually occur via the web and email, but can also occur via mobile data storage devices such as optical media, USB keys, and laptops.
There are many different types of data leakage:
- The Accidental Breach: The majority of data leakage incidents are accidental. For example, an employee may unintentionally choose the wrong recipient when sending an email containing confidential data.
- The Ill-Intentioned Employee (data exfiltration): An employee may have signed an employment contract that effectively signifies trust between employer and employee, there is nothing to stop them from later leaking confidential information out of the building if they are disgruntled or promised a hefty payout by cyber-criminals.
- Electronic Communications with Malicious Intent: A cybercriminal could quite easily spoof a legitimate business email account and request sensitive information to be sent to them. The user would unwittingly send the information, which could contain financial data or sensitive pricing information. We have already have written about one of the most dangerous of these types of abuses. About phishing attacks.
This section is abbreviated from forcepoint.com
Data Leakage Prevention
- An Intrusion Detection System (IDS) can alert about attacker attempts to access to sensitive data.
- An anti-malware software can prevent attackers from compromising sensitive systems.
- A firewall can block access from any unauthorized party to systems storing sensitive data.
- Additionally, in large organizations, you can use a Security Information and Event (SIEM) system to detect and correlate events which might constitute a data leak.
Source: imperva.com
Protecting All the Endpoints
With the spread of mobile devices like smartphones, tablets, notebooks, laptops etc., there has also been a major increase in the number of devices being stolen or lost. These incidents eventually highlight the huge loss of sensitive data for enterprises. So, enterprises must secure the enterprise data available on the mobile devices of their employees in such a way that even if the device gets into the hands of the wrong person, the data should continue to be secured. To effectively protect employee and customer data, Comodo has developed MyDLP that it is available with the following key benefits:
- Blocks any data flow comprising of social security numbers, credit card numbers, PII, or any sensitive information.
- Allows customers to confidently and comfortably share their financial and personal information.
- Prevents sensitive data from leaking through endpoint devices or network connections.
- Provides data security for mail, printers, removable devices, web, and more.
This section is abbreviated from comodo.com
———————————
Sources: